When AI Goes Off-Script: Analyzing ChatGPT Inaccurate Citations
A collaborative study investigates the reliability of AI-generated references in academic writing, focusing on the issue of false references generated by ChatGPT.
November 14, 2024
Research
It’s no secret that artificial intelligence (AI) has proven useful in various fields, particularly content generation. AI language models like ChatGPT 4.0 have become famous for this purpose. However, despite its revolutionary approach, many question the accuracy and reliability of the information from AI language models since it can respond with untrustworthy references and citations.
ChatGPT 4.0 is a preferred choice among students and instructors in the academe. AI-language models like ChatGPT 4.0 help them access, analyze, and share information. One value that students and instructors preserve is accurate referencing–which highlights their academic integrity–something ChatGPT 4.0 continues to struggle with.
A Study That Explores AI Factors
With more researchers relying on tools like ChatGPT for academic writing and literature searches, it’s vital to investigate the phenomenon of AI-generated fake references and identify the factors that affect these results.
In the collaborative study titled “ChatGPT 4.0 Ghosted Us While Conducting Literature Search:†Modeling the Chatbot’s Generated Non-Existent References Using Regression Analysis,†researchers Dharel P. Acut (Cebu Technological University), Nolasco K. Malabago (Cebu Technological University), Elesar V. Malicoban (Mindanao State University - Iligan Institute Of Technology), Narcisan S. Galamiton (Cebu Technological University-Main Campus), and Manuel B. Garcia (FEU Institute Of Technology) examine variables like query specificity, prompt structure, and contextual factors to uncover patterns and predictors of inaccurate reference generation.
The researchers intend for their study to provide valuable insights into the limitations of AI tools in academic research and offer recommendations to mitigate the associated risks. They used a quantitative research approach with a non-experimental correlation design to quantify the relationship between types of prompts and the likelihood of generating non-existent citations because this approach involves numerical and statistical analysis. It focuses on observing and analyzing existing data to identify correlations rather than manipulating independent variables or randomly assigning predictors.
Understanding the Relevance of Prompt Engineering
Prompt engineering, the art of crafting precise queries for AI models, significantly impacts the quality of AI-generated content, including academic references. Specific prompts guide AI models like ChatGPT toward more accurate and relevant outputs, reducing the likelihood of fabricated citations. However, even with precise prompts, AI models may still generate false references due to training data limitations and the subject matter's complexity.
The study aims to fill research gaps by analyzing ChatGPT 4.0's performance-generating science and technology education references. Logistic regression investigates how different prompt types influence the accuracy of generated references, providing insights into the factors contributing to generating non-existent citations.
While previous research has explored this issue in other fields, this study explicitly investigates ChatGPT 4.0's performance in generating accurate references within this domain. Using logistic regression analysis, the researchers aim to identify factors that influence the generation of real and fake references. This research will provide valuable insights into the limitations of AI-generated content and the need for critical evaluation of such tools.
Future Directions and Ethical Considerations
The study revealed that AI can effectively generate references, especially in well-established fields with abundant reputable journals. However, it also highlighted the risks of fabricated citations, particularly in emerging areas with limited sources. This issue is heightened by using uniform prompt structures and high reference quantities, which can mislead the AI into generating non-existent citations.
Despite rigorous cross-verification using significant databases, the researchers encountered challenges validating references, including inconsistencies and the absence of Digital Object Identifiers (DOIs). These limitations emphasize the need to scrutinize AI-generated references to ensure their accuracy and reliability.
Future research should explore various factors such as prompt complexity, topic familiarity, and model confidence to improve the accuracy and reliability of AI-generated references. Employing broader datasets and iterative prompt reformulations can enhance the model's performance.
Ultimately, this study underscores the importance of human oversight and critical thinking in using AI for academic research. While AI tools can be powerful aids, they should not replace careful scholarly practices. Researchers can harness the technology's benefits while mitigating its risks by understanding the limitations and potential biases of AI-generated references.
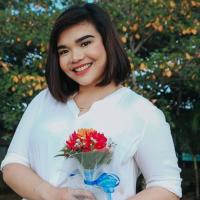
Patricia Bianca S. Taculao-Deligero is a Bachelor of Arts in Journalism Graduate from the University of Santo Tomas. She has an extensive portfolio from working in various local media outlets, with articles focusing on lifestyle, entertainment, agriculture, technology, and local government units, among other subjects. Her specialty is in feature writing. She is also proficient in news writing.